Subtitling, automation and machine translation
a teaching and learning experience
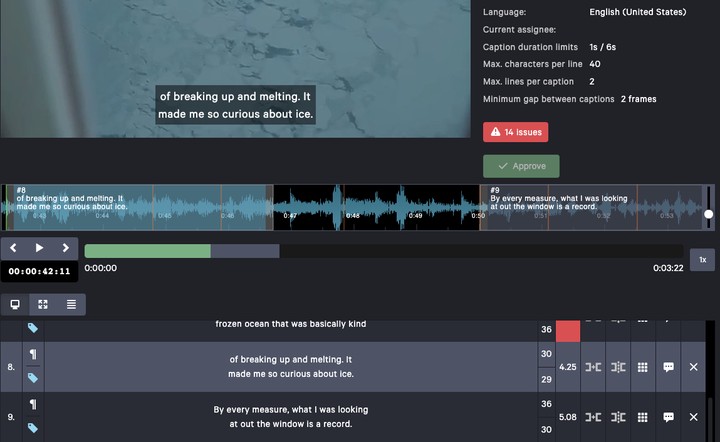
As a visiting lecturer at the University of Warsaw, between April and July 2022, I was teaching the course Subtitling, Automation and Machine Translation. The course (an elective MA module) allowed me to develop and deliver an innovative course on a subject that was also new to me: AI-enhanced subtitling.
This is a short reflection on that experience and some lessons I learned through this course which I intended to make socially responsible, flexible and industry relevant.
There are two main reasons why I think this type of courses will only become more integral in translator training:
- The translation profession is changing so quickly that we, as trainers, are regularly required to teach new skills, many of which are emerging only now and, consecutively, might be unfamiliar to us.
- Making students aware of these regular changes will (I hope) help them break into the market and create sustainable careers for themselves.
In line with the steady expansion of the language industry and the claims of a talent crunch, I was particularly interested in teaching this module. I expected it would provide me with the experience to assess how academia can respond faster to the changing needs of companies, translators and society, and how we, as trainers, can ensure we address the demand to foster completely new skills among our students.
Background and personal challenges
The course was initially entitled “Subtitling and post-editing” in February 2020 but was delayed due to the pandemic. In the meantime, discussions on wider societal issues of AI and automation gained traction in the NLP and MT communities: language inequality, bias in language data and models, working conditions… As a result, I broadened the focus of the course to account for these developments.
Course description
The course explored the relationship between subtitling and automation, with a focus on automatic transcription, automatic spotting, and machine translation. I wanted to provide students with theoretical knowledge to understand and assess how subtitling practices and the profession are being changed by automation. Building on this foundation, I wanted students to develop practical skills to critically evaluate the benefits and shortcomings of machine translation and, more generally, automation as applied to subtitling.
Ultimately, the learning objectives of the course were for students to be able to:
- Understand the systems and their impact on their work and the translation profession
- Critically assess different MT and ASR systems under different conditions
- Design their own workflows, integrating different ASR and MT tools depending on their needs, requirements and affordances.
Integrating new skills
Since the module involved new skills and tools that are constantly developing, my main challenge was to find a way to teach skills and competences that I needed to acquire and improve myself. When it comes to subtitling, post-editing and automation are only now being adopted in workflows. Training resources are limited and the tools as such also change regularly. Thus, the course demanded a flexible, responsive and adaptable curriculum.
I framed the course and the development of these skills as a problem-solving exercise. Problem-solving is essential to any language professional and by approaching the development of skills and the adoption of new tools as part of a core-professional skill, I tried to establish a bridge between the new tools and the students’ existing skillset. For a while now, I have been implementing a similar approach to my technology classes (see Troubleshooting guide below) in an attempt to encourage students to be active agents in their learning process and act proactively in finding solutions to the challenges they face.
For each task (transcription, spotting, translating for subtitling and QA), students needed to test different tools as a way to become familiar with them, assess their performance and make an informed decision about their usability.
I encouraged students not only to learn how to use the tools but regularly evaluate their usability, functionality and added value.
To address the broader issues regarding the industry and the market, I relied on invited speakers. The guests shared their expertise and knowledge with the group. This allowed me to make sure students could learn from experts from different sectors and academic disciplines. I was very thankful that colleagues in the industry, computer science and subtitling research accepted the invitation:
- Yota Georgakopoulou, Athena Consultancy
- Alina Karakanta, Fondazione Bruno Kessler
- Samuel Läubli, TextShuttle, ZHAW
- Anke Tardel, Johannes Gutenberg University Mainz
Content
The course was divided into seven sessions and covered these topics:
- Automation and its application to subtitling
- Principles and consequences of neural machine translation
- Speech transcription and automatic spotting
- Post-editing for subtitling
- Subtitling software integrating post-editing
- Subtitling workflows that involve post-editing and automation
- Ethical, social and professional implications of MT
Tools
- YouTube
- CaptionHub
- MateSub
- AppNet
- ModelFront
- Google Translate
- DeepL
Class activities
The tasks were incremental and students used a different tool at every step to be able to compare different results:
- transcription and spotting: YouTube and CaptionHub
- intralingual and interlingual subtitling: CaptionHub
- comparing MT: CaptionHub, MateSub, AppTek
- independent project: any MT engine and subtitling software
- Examples of additional MT features: ModelFront, Lilt
What we learned
Making students aware of the purpose of the activities and empowering them to assume a critical stance when using the tools helped create a collaborative environment. A constant challenge in the classroom was emphasising that AI and automated solutions (in the form of transcription, spotting or translation) should not be expected to produce perfect results.
Monitoring actions are essential in post-editing to achieve publishable quality results.
This human-in-the-loop approach places value on the integration of technological tools and human knowledge and skills. I stressed that the goal was to assess the benefits of integrating different tools into professional workflows, while still recognising that revising the automatic transcription and the machine translation output, as well as conducting thorough QA processes, constitute an essential part of the process.
Roughly, the main stages in the process were defined as indicated in the graph below. Students were encouraged to modify these activities as needed for every task and depending on the tools used.
Overall, the subtitlers were impressed with the quality of the automatic transcription. Many expected to see previous known issues, such as accents and audio quality, but were surprised to see these seem to be less problematic with current systems. Automatic speaker identification, when correct, was also mentioned as a useful resource.
Students commented on the advantage of speeding up the transcription and spotting processes. They noticed the way in which transcription and spotting are managed also varies across tools. In the case of CaptionHub, the automatically transcribed text is immediately spotted and turned into subtitle blocks. On the contrary, MateSub offers transcription in two ways: a timed word-level transcription and transcription into subtitles.
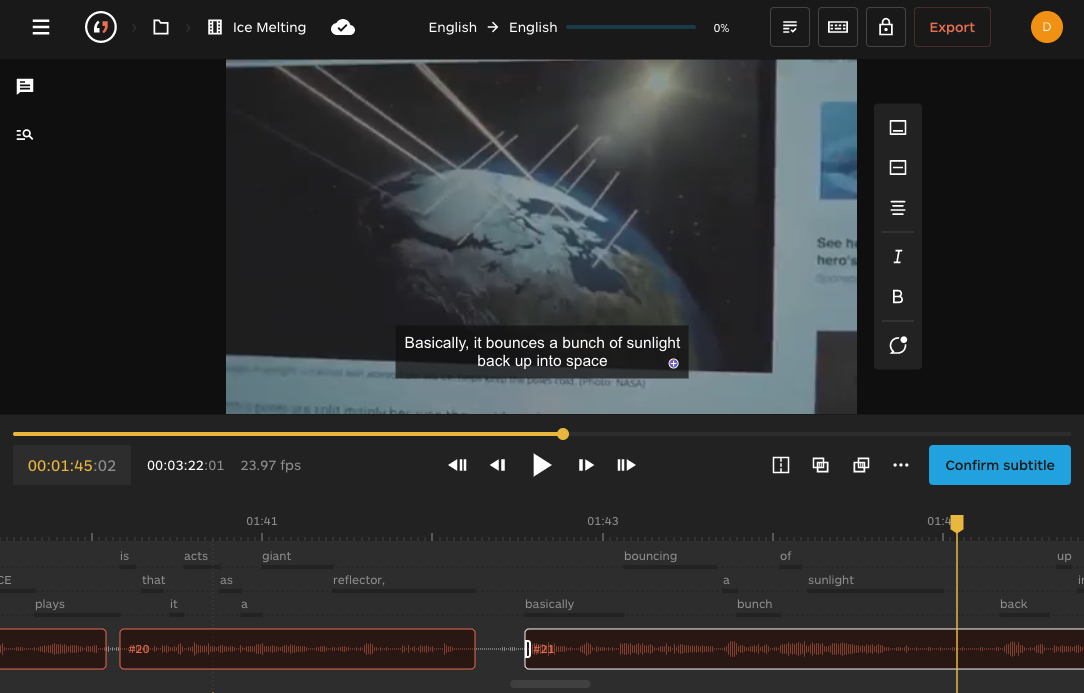
Matesub
While students found spotting generally useful, automatic segmentation was seen as more problematic, as not all systems integrate subtitling-specific segmentation rules.
Combining automatic transcription and MT
After the initial tasks and testing of different MT systems, students commented that the human revision of the automatic transcription did not necessarily contribute to increasing the quality of the MT output. Thus, if the aim was not to generate source-language subtitles, many opted for skipping the revision of transcription and spotting. They decided to focus on full post-editing of the MT and a final review of the target-language subtitles.
Both CaptionHub and MateSub include pre-defined subtitling guideline features (number of lines, characters per line, etc.). Students mentioned this is an indication that the systems were prepared to manage different needs and recognise the variation across sectors and clients in the market.
Adapting post-editing guidelines to subtitling
As a way of tailoring post-editing to subtitling workflows, we reflected on how the TAUS post-editing guidelines could be adapted to subtitle post-editing.
According to class discussions, some aspects that need to be integrated into the TAUS guidelines to make them suitable for subtitle PE are:
Light PE
- Checking for missing transcriptions
- Making sure subtitles are unambiguous
- Verifying spelling for proper nouns and names
- Fixing extremely fast subtitles
- Adding or correcting speaker indicators, such as dashes in dialogues
- Revise false positive subtitles (subtitles created when there is no speech)
Full PE
- Revise spotting
- Applying condensation strategies as needed to improve subtitle speed
- Correcting punctuation and spacing issues
- Correct segmentation: adequate distribution between the two lines and subsequent subtitles
- Merging short subtitles and splitting long subtitles, as needed
When pre-editing is used to create same-language subtitles, then the process should consider similar aspects as those listed above.
Improving tool functionality
Since many of the students had previous training in subtitling or professional experience as subtitlers, some found using tools such as CaptionHub and Matesub limiting. In a way, this experience exemplifies the issues involved in challenging routine expertise: students were already used to using subtitling software that offered them more advanced functionalities, such as EZTitle and OOONA. Thus, apart from adapting to the post-editing task, the students also needed to adapt themselves to the tools.
Some of the features students missed the most were:
- Automatic subtitle chaining
- Moving words from a subtitle to another
- Lack of display personalisation options
- Limited spell-checking options
- Restrictions in using and creating key combinations
Apart from this, students also mentioned that it would be useful to integrate other translation tools, such as translation memories and concordancers. In the case of MT, as is the case with other implementations of MT in CAT tools, integrating quality estimation information could also support the post-editing process.
Testing different platforms and tools in the same course allows students to understand hardware implications as well. Some ergonomics considerations emerged through the evaluation of the tools since students faced issues with the size of their screens and their keyboard distributions.
Final remarks
The feedback from students was very positive, with the majority of them commenting they feel confident they can efficiently evaluate and implement automated solutions into their subtitling workflows.
Helping students become aware of their role in exploring and assessing the tools provided them with the opportunity to tailor the tasks to their own workflows and preferences. This could help enhance problem-solving and adaptability, thus reducing functional fixedness and leading students to develop skills and attitudes that will help them create sustainable career paths.